How to Use AI to Detect Fraudulent Transactions in E-Commerce
Vimal Tarsariya
May 12, 2025
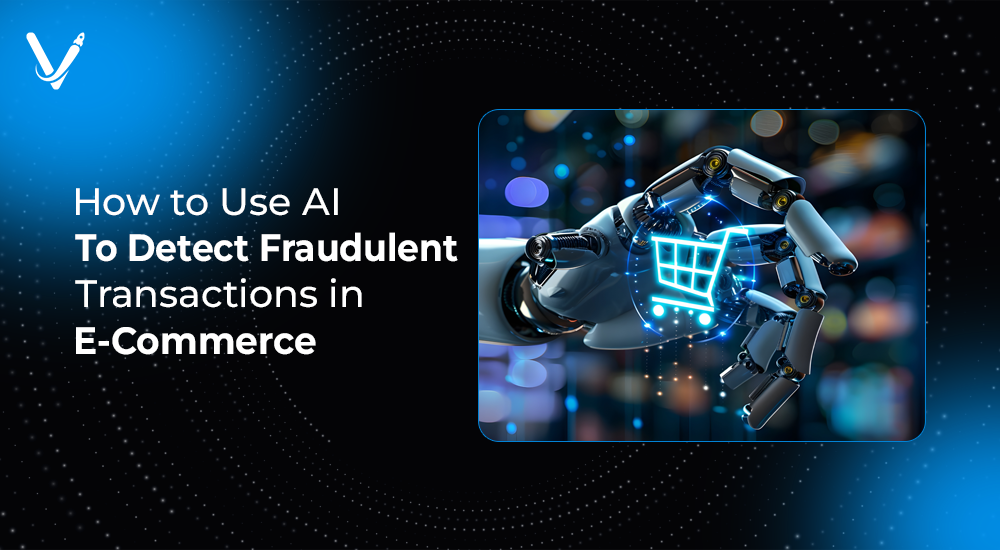
E-commerce has revolutionized the way businesses and consumers interact, enabling seamless transactions across the globe. However, as online transactions surge, so do fraudulent activities. Fraudulent transactions not only result in financial losses but also damage brand reputation and erode customer trust.
This is where Artificial Intelligence (AI) in e-commerce steps in. AI-powered systems are capable of identifying fraudulent activities in real-time by analyzing massive datasets, detecting patterns, and predicting potential threats. In this article, we explore how AI is being utilized to detect fraudulent transactions in e-commerce, the types of fraud it can prevent, best practices for implementing AI-based fraud detection systems, and real-world examples of AI in action.
Understanding E-Commerce Fraud and Its Impact
E-commerce fraud encompasses a range of deceptive activities aimed at exploiting online payment systems. These fraudulent transactions often involve the unauthorized use of payment information, chargebacks, account takeovers, and refund fraud.
Common Types of E-Commerce Fraud:
- Card Not Present (CNP) Fraud: Fraudsters use stolen credit card details to make unauthorized purchases.
- Account Takeover (ATO) Fraud: Hackers gain access to customer accounts, altering payment information to execute fraudulent transactions.
- Chargeback Fraud: Customers dispute legitimate transactions, claiming they did not receive the product or service.
- Refund Fraud: Fraudsters exploit return policies to receive refunds for items they did not purchase.
- Triangulation Fraud: Fraudsters create fake online stores, collect customer payment information, and use it to make fraudulent purchases.
The financial impact of e-commerce fraud is staggering. According to a report by Juniper Research, global e-commerce fraud losses are expected to reach $48 billion by 2025, emphasizing the urgent need for robust fraud prevention strategies.
How AI Detects Fraudulent Transactions in E-Commerce
AI leverages advanced algorithms, machine learning (ML), and data analytics to detect fraudulent transactions in e-commerce. By analyzing transactional data in real time, AI can identify anomalies and flag suspicious activities before they escalate.
How AI Works in Fraud Detection:
- Data Collection and Analysis: AI systems collect data from multiple sources, including transaction history, payment methods, customer behavior, and device information.
- Pattern Recognition: AI identifies patterns associated with fraudulent transactions, such as unusual purchase amounts, high-risk geolocations, and rapid transaction frequencies.
- Anomaly Detection: AI compares current transactions against historical data, highlighting discrepancies that may indicate fraud.
- Predictive Analytics: AI algorithms predict potential fraud based on past patterns, enabling proactive measures.
- Risk Scoring: AI assigns a risk score to each transaction, categorizing it as low, medium, or high risk.
- Real-Time Alerts: AI systems generate real-time alerts, notifying fraud analysts of potential threats.
AI-Powered Fraud Detection Techniques in E-Commerce
1. Machine Learning Models for Fraud Detection
Machine learning models are at the core of AI-based fraud detection systems. These models are trained using historical data to identify patterns and detect anomalies.
- Supervised Learning: The model is trained on labeled datasets containing both fraudulent and legitimate transactions. Once trained, the model can predict the likelihood of fraud in new transactions.
- Unsupervised Learning: Unsupervised models analyze unlabeled data to detect anomalies, making them effective in identifying emerging fraud patterns.
- Reinforcement Learning: These models learn from real-time feedback, continuously updating their algorithms to improve detection accuracy.
Example:
PayPal uses machine learning to analyze transaction data and detect fraudulent activities in real time. The system considers factors such as purchase amount, device ID, and transaction location to flag suspicious behavior.
2. Neural Networks and Deep Learning
Neural networks simulate the human brain, processing vast datasets to identify complex patterns associated with fraud. Deep learning algorithms can detect subtle correlations that traditional systems may miss.
- Convolutional Neural Networks (CNNs): Effective in analyzing image data, such as identifying fraudulent receipts or invoices.
- Recurrent Neural Networks (RNNs): Useful in detecting sequential patterns in transactional data, such as recurring chargeback fraud.
- Long Short-Term Memory Networks (LSTMs): Identify time-based patterns, such as multiple transactions from a single IP address within a short timeframe.
3. Natural Language Processing (NLP) in Fraud Detection
NLP analyzes textual data to detect fraudulent activities, such as phishing emails, fake reviews, or suspicious customer messages.
- Sentiment Analysis: Detects negative sentiments in customer complaints, indicating potential fraud.
- Text Mining: Analyzes communication data for fraud indicators, such as keywords related to chargebacks or refunds.
Example:
Amazon uses NLP to monitor customer feedback and identify fraudulent sellers who manipulate reviews to boost product ratings.
Implementing AI in E-Commerce Fraud Detection: Best Practices
1. Data Integration and Centralization:
Consolidate data from multiple sources, including transaction history, payment gateways, and customer profiles, to provide AI systems with comprehensive datasets.
2. Continuous Model Training:
Regularly update AI models with new data to keep pace with evolving fraud tactics. Implement feedback loops to refine algorithms based on detected fraud.
3. Multi-Layered Security Approach:
Combine AI with other security measures, such as biometric authentication, encryption, and secure payment gateways.
4. Real-Time Monitoring:
Implement AI systems that monitor transactions in real time, flagging suspicious behavior immediately.
5. Regulatory Compliance:
Ensure AI systems comply with data protection regulations, such as GDPR and PCI DSS, to maintain data security and customer trust.
Real-World Examples of AI in E-Commerce Fraud Detection
1. PayPal:
PayPal uses AI algorithms to analyze billions of transactions, identifying fraudulent activities by comparing transaction data against known fraud patterns.
2. Amazon:
Amazon employs AI to detect fraudulent sellers, prevent account takeovers, and flag suspicious payment activities.
3. Stripe:
Stripe leverages AI-powered fraud detection tools to monitor transactions, assigning risk scores and blocking high-risk payments.
The Future of AI in E-Commerce Fraud Detection
The future of AI in e-commerce fraud detection will involve more sophisticated algorithms capable of detecting emerging fraud tactics. Predictive analytics will enable e-commerce platforms to anticipate fraud before it occurs, while blockchain technology will provide immutable transaction records, enhancing transparency.
Conclusion
AI is revolutionizing fraud detection in e-commerce, providing businesses with powerful tools to detect, prevent, and mitigate fraudulent activities in real time. By leveraging advanced algorithms, machine learning, and predictive analytics, AI-powered fraud detection systems can identify subtle fraud patterns, mitigate risks, and safeguard customer data.
At Vasundhara Infotech, we specialize in implementing AI-based fraud detection solutions tailored to e-commerce platforms. Ready to protect your business against fraud? Contact us today to learn more about how AI can secure your transactions and enhance customer trust.